Reinforcement Learning Training Ai Through Trial And Error
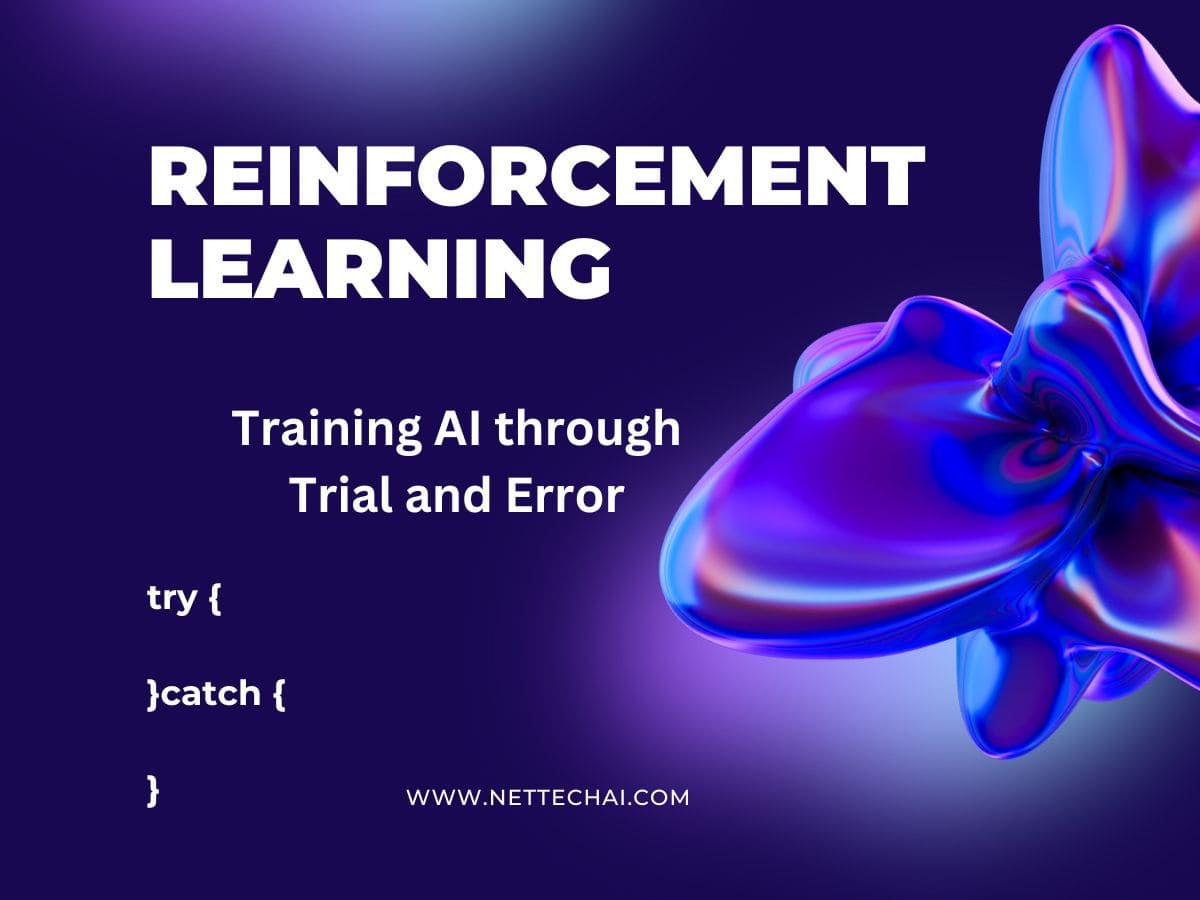
In the quest to bestow artificial intelligence (AI) with the ability to learn and adapt, Reinforcement Learning emerges as a pivotal paradigm. This article embarks on a comprehensive exploration of Reinforcement Learning, unraveling its essence, dissecting its components, investigating its real-world applications, and peering into the challenges and future prospects that shape its transformative potential.
Reinforcement Learning (RL) Explained
Reinforcement Learning is a dynamic subfield of AI that centers around the idea of training agents to make a sequence of decisions by interacting with their environment. Unlike traditional supervised learning, where labeled examples guide learning, RL hinges on agents navigating their surroundings through trial and error to optimize a reward signal. This approach mirrors how humans learn by experiencing the world around them.
How Agents Learn from Interaction
Reinforcement Learning operates under the premise of an agent interacting with an environment. The agent takes actions, leading to changes in the environment and yielding rewards or penalties. The agent’s objective is to learn a policy—a strategy for selecting actions—that maximizes cumulative rewards over time. Through continuous interaction, the agent refines its policy to make better decisions in various scenarios.
Components of Reinforcement Learning
Rewards, actions, and states form the core components of Reinforcement Learning.
- Rewards serve as the feedback mechanism that guides the agent’s learning. Positive rewards reinforce desired behaviors, while negative rewards discourage undesirable actions.
- Actions are the choices an agent can make to interact with the environment, influencing subsequent states and rewards.
- States represent the environment’s condition at a particular point in time, encompassing the information necessary for decision-making.
Exploration vs. Exploitation Dilemma
A fundamental challenge in Reinforcement Learning is the exploration vs. exploitation trade-off. While exploitation entails selecting actions that yield known rewards, exploration involves trying new actions to discover potentially better strategies. Striking the right balance between exploration and exploitation is crucial for agents to acquire optimal policies, as solely focusing on known actions can prevent the discovery of better strategies.
Real-World Applications
It’s applications extend across diverse domains, offering solutions to complex challenges.
In the realm of robotics and automation, Reinforcement Learning equips robots to learn tasks through repeated interactions. Robots can learn to navigate complex environments, manipulate objects, and adapt to dynamic situations. Game-playing AI agents showcase RL prowess by mastering intricate games like Go, Chess, and video games. These agents learn strategies through gameplay and competition against human players. In the realm of self-driving cars, RL enables vehicles to navigate roads, make decisions, and respond to real-time traffic conditions, fostering safer and more efficient transportation.
Challenges and Future Prospects
Reinforcement Learning presents a series of challenges that continue to drive research and innovation.
Handling Complex Environments
Reinforcement Learning encounters difficulties in scaling to complex environments with vast action spaces and intricate dynamics. Training agents in such environments often requires extensive time and resources. Research efforts are directed toward developing techniques that enhance the efficiency of learning in complex scenarios.
Combining Reinforcement Learning with Other AI Techniques
To address challenges, researchers are exploring hybrid approaches that combine RL with other AI techniques. For instance, integrating Reinforcement Learning with imitation learning enables agents to learn from demonstrations provided by human experts. This approach accelerates learning and reduces the time required for agents to acquire effective policies.
Looking Ahead
Its trajectory promises transformative advancements in AI.
Multi-Agent Reinforcement Learning
The future holds the potential for multi-agent RL, where multiple agents learn and interact with one another. This paradigm has implications for understanding cooperative and competitive behaviors, enabling the creation of AI systems that collaborate in complex scenarios.
Real-World Impact
Reinforcement Learning’s impact extends beyond research laboratories to real-world applications that enhance automation, transportation, and decision-making. As algorithms become more efficient and agents acquire more diverse experiences, the potential for RL to revolutionize industries becomes more apparent.
In conclusion, It represents a fundamental shift in AI paradigms, enabling agents to learn and adapt through trial and error. Its components, such as rewards, actions, and states, lay the groundwork for agents to navigate complex environments. It’s applications span robotics, gaming, and transportation, demonstrating its versatility and potential. As challenges are addressed and new approaches emerge, the future holds the promise of more sophisticated and impactful RL systems. Through this dynamic interplay of learning and adaptation, Reinforcement Learning fuels AI’s journey toward more intelligent and capable systems.